As therapies for cancer become more available world-wide, there is a need to develop screening tools to diagnose potential patients overcoming geographical and economic limitations. In order to improve the quality of patient categorization and diagnosis, the Diagnostic Intelligent Assistance for Global Health (DIAG) team seeks students to join us on two main projects that will develop software-based tools to facilitate diagnosis and interpretation of cancer image data.
- Artificial Intelligence-enabled Diagnostics (AID): Students will develop image-based screening tools for rapid disease categorization and diagnosis support. The AID tool is envisioned as an AI-based image analysis tool, deployed on the cloud that will enable disease categorization and diagnosis regardless of economic and geographical constraints. With an eye towards Low- and Medium-Income Countries (LMIC), this tool will support clinical users who upload standard-of-care histology images to the cloud and obtain rapid feedback to support their diagnoses, even in scenarios where the accessibility to expert pathologists is limited. This project will draw students from EECS, CS/CE, BME and just about any major interested to contribute to the goal of bringing AI-enabled quality clinical decision-making to everyone on this planet.
- Spatial Analytics for the Cancer Ecosystem (SPACE): Using modern tools from environmental monitoring, climatology, space sciences and geographical AI; we aim to fundamentally rethink the interpretation of cancer imaging data for better diagnostics. The SPACE project will integrate geographical and spatial modeling tools with AI methods like deep learning. We invite all non-linear thinkers coming from various disciplines as diverse as quantitative geography, environmental monitoring and even climate/disaster modeling to work alongside machine learning/AI enthusiasts to build a new paradigm. Students from all engineering disciplines interested in applying spatial modeling ideas to interpreting biomedical image data are welcome.
Team Organization
This team has flexible subteams that allow students to deepen their learning for the AID and SPACE projects. The project teams will draw from roles such as System Design, Data Engineering, Modeling and AI, as well as Software Design and Prototyping. Each subteam will also have a student team leader that reports to the faculty PI and is supported by other doctoral students in the lab.
First-year undergraduates through master’s graduate students are welcome to apply, and all will be encouraged to stay on the team for more than the two-semester minimum. Leadership roles are available in the lab, and experienced students will be a natural fit for these positions as their knowledge grows over time.
Below are the skills needed for this project. Students with the following relevant skills and interest in the project are encouraged to apply! Although the team consists of subteams, students apply to the project as a whole, rather than individual roles on the team.
System Design (3 Students)
Preferred Skills: Systems & Engineering Thinking
Likely Majors: EECS, CS, CE, BME, IOE, PUBHLTH, ANY
Data Engineering, Modeling and AI Design (5-7 Students)
Preferred Skills: Computer Vision, AI, Machine Learning, image processing, general programming, Data Sciences
Likely Majors: EE, CS, BME, SI, DATA
(for the SPACE project, any majors interested in spatial modeling, including students from Environmental Science, School for Environment and Sustainability (SEAS), Program in the Environment (PiTE), Space Science, Climate & Meteorology are welcome to apply)
Software Design & Prototyping (3 Students)
Preferred Skills: Developing user-interfaces
Likely Majors: CS, SI, ANY
Apprentice Researchers (3 Students)
Requirements: Interest in project material, willingness to develop skills. Students will be integrated into the operations of a subteam. Open to first- and second-year undergraduate students ONLY.
Likely Majors: ANY
All students will be required to complete PEERRS human subject training before beginning work.
Faculty Sponsor
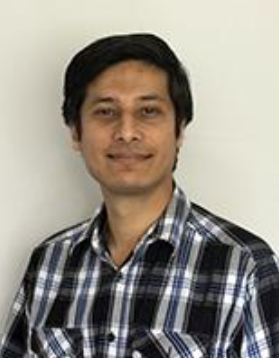
Arvind Rao, PhD
Associate Professor, Dept of Computational Medicine & Bioinformatics
Associate Professor, Radiation Oncology
Arvind Rao is an Associate Professor in the Department of Computational Medicine and Bioinformatics, Radiation Oncology, Biostatistics and an affiliate association with Biomedical Engineering at the University of Michigan. Arvind received his PhD in Electrical Engineering and Bioinformatics from the University of Michigan, specializing in transcriptional genomics, and was a Lane Postdoctoral Fellow at Carnegie Mellon University, specializing in image informatics. He was previously on the faculty in Bioinformatics and Computational Biology at the University of Texas MD Anderson Cancer Center. His research group uses image analysis and AI/machine learning methods to mine and integrate different kinds of data sources for health applications like drug repurposing, spatial systems biomedicine, imaging diagnostics and health informatics. His interest in Single Cell Spatial Analysis involves developing bioinformatics and data science approaches for the interpretation and integration of data from spatial transcriptomics, proteomic & imaging platforms.
Weekly Meeting Time and Location: MDP Team meetings (all members expected to attend) are Tuesdays from 4:00 pm – 5:30 pm ET on Zoom. Each subteam also arranges at least separate convenient weekly time to meet and work together following university guidelines. A two-term commitment will begin January 2024.
Course Substitutions: Honors, CS-ENGR/DS-ENGR/EE/CE-ENGR 355 and higher can count toward Flex Tech
These substitutions/departmental courses are available for students in these respective majors. MDP does not yet have a formal agreement with other departments for substitutions/departmental courses not listed. Please reach out to your home department’s academic advisor about how you might apply MDP credits to your degree plan.
Citizenship Requirements: This project is open to all students on campus
IP/NDA: Students who successfully match to this project team will be required to sign an Intellectual Property (IP) Agreement prior to participation in January 2024.
Summer Opportunity: Summer research fellowships may be available for qualifying students
Learn more about the expectations for this type of MDP project